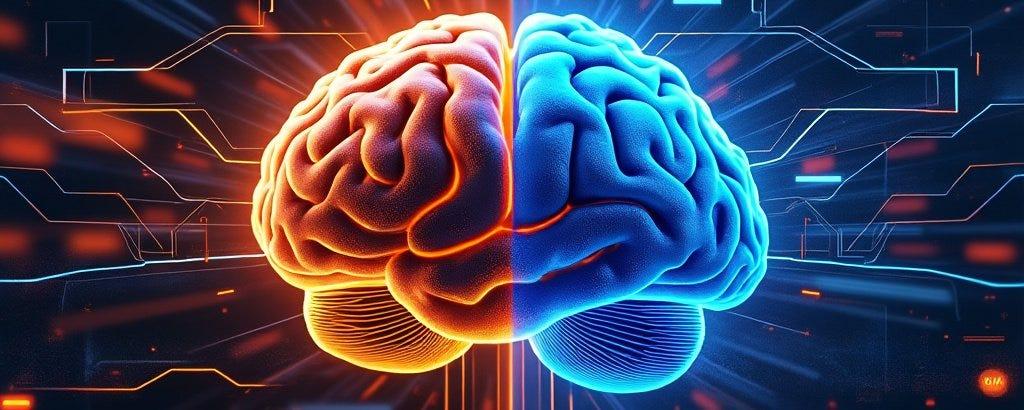
Thanks to: @chiefbuidl whose insights provoked me to think deeper about challenges for Web 3.0 agents; @TheoriqAI that sets the blueprint for projects in this direction; @jonathankingvc whose curiosity motivated me to write this post.
The Promise of Centralized AI Agents
AI agents have the potential to revolutionize how we interact with the web and perform tasks online. While there’s been significant buzz around AI agents leveraging crypto payment rails, it’s important to recognize that established Web 2.0 companies are also well-positioned to offer a comprehensive suite of agent products. Tech giants like Apple, Google, and AI specialists such as OpenAI or Anthropic seem particularly well-suited to explore synergies in developing autonomous agent systems.
Apple’s strength lies in its ecosystem of consumer devices, which could serve as both hosts for AI models and gateways for user interaction. The company’s Apple Pay system could enable agents to facilitate secure online payments. Google, with its vast index of web data and capability to provide real-time embeddings, could offer agents unprecedented access to information. Meanwhile, AI powerhouses like OpenAI and Anthropic could focus on developing specialized models capable of handling complex tasks and managing financial transactions.
However, these Web 2.0 giants face a classic innovator’s dilemma. Despite their technological prowess and market dominance, they must navigate the dangerous waters of disruptive innovation. The development of truly autonomous AI agents represents a significant departure from their established business models. Moreover, the unpredictable nature of AI, coupled with the high stakes of financial transactions and user trust, presents significant risks.
The Innovator’s Dilemma: Challenges for Centralized Providers
The innovator’s dilemma describes a paradox where successful, established companies often struggle to adopt new technologies or business models, even when these innovations are crucial for long-term success. The core of the problem lies in the reluctance of incumbent firms to introduce new products or technologies that initially offer a poorer user experience compared to their existing, refined offerings. These companies fear that embracing such innovations might alienate their current customer base, who have come to expect a certain level of polish and reliability. This hesitation comes from the risk of disrupting the carefully cultivated expectations of their users.
Unpredictable Agents and User Trust
Large tech companies like Google, Apple, and Microsoft have built their empires on tried-and-true technologies and business models. Introducing fully autonomous AI agents represents a significant departure from these established norms. These agents, especially in their early stages, will inevitably be imperfect and unpredictable. The non-deterministic nature of AI models means that even with extensive testing, there’s always a risk of unexpected behavior.
For these companies, the stakes are incredibly high. A misstep could not only damage their reputation but also expose them to significant legal and financial risks. This creates a strong incentive to move cautiously, potentially missing out on the first-mover advantage in the AI agent space.
The risk of customer backlash looms large for centralized providers considering AI agent deployment. Unlike startups that can pivot quickly and have less to lose, established tech giants have millions of users who expect consistent, reliable service. Any significant failure of an AI agent could lead to a public relations nightmare.
Consider a scenario where an AI agent makes a series of poor financial decisions on behalf of users. The resulting outcry could potentially erode years of carefully built trust. Users might question not just the AI agent but all of the company’s AI-powered services.
Ambiguous Evaluation Criteria & Regulatory Challenges
Moreover, the ambiguity in assessing what constitutes a “proper” agent response further complicates matters. In many cases, it may be unclear whether an agent’s action was truly erroneous or simply unexpected. This gray area could lead to disputes and further damage customer relationships.
Perhaps the most daunting obstacle for centralized providers is the complex and evolving regulatory landscape surrounding AI agents. As these agents become more autonomous and handle increasingly sensitive tasks, they enter a regulatory gray area that could pose significant challenges.
Financial regulations are particularly thorny. If an AI agent is making financial decisions or executing transactions on behalf of users, it may fall under the purview of financial regulators. The compliance requirements could be extensive and vary significantly across different jurisdictions.
There’s also the question of liability. If an AI agent makes a decision that results in financial loss or other harm to a user, who is held responsible? The user? The company? The AI itself? These are questions that regulators and lawmakers are only beginning to grapple with.
Model Bias as a Source of Dispute
Furthermore, as AI agents become more sophisticated, they may bump up against antitrust regulations. If a company’s AI agents consistently favor that company’s own products or services, it could be seen as anti-competitive behavior. This is especially relevant for tech giants that already face scrutiny over their market dominance.
The unpredictable nature of AI models adds another layer of complexity to these regulatory challenges. It’s difficult to guarantee compliance with regulations when an AI’s behavior can’t be fully predicted or controlled. This unpredictability could lead to slower progress in the Web2 space as companies navigate these complex issues, potentially giving an advantage to more agile Web3 solutions.
The Web3 Opportunity
Web3 technologies offer unique opportunities for AI agent development that could potentially address some of the challenges faced by centralized providers. Crypto-economic incentives can facilitate agent discovery and provide a stake that can be slashed if agents misbehave. This creates a self-regulating system where good behavior is rewarded and bad behavior is punished, potentially reducing the need for centralized oversight and providing some level of comfort for early adopters that entrust financial transaction execution to fully autonomous agents.
Crypto-economic stakes have a twofold role being required for slashing in case of misbehavior, as well as being used as a critical market signal in the process of discovering AI agents, whether by other agents or humans seeking specific services. The intuition is straightforward, the more stake the more market trust in the performance of particular agents, the more peaceful state of mind of the users. This could create a more dynamic and responsive ecosystem of AI agents, where the most effective and trustworthy agents naturally rise to the top.
Web3 also enables the creation of open marketplaces for AI agents. These marketplaces allow for greater experimentation and innovation compared to trusting centralized providers. Startups and independent developers can contribute to the ecosystem, potentially leading to more rapid advancement and specialization of AI agents.
Additionally, distributed networks like Grass and OpenLayer can provide AI agents with access to both open internet data and gated information that requires authentications. This broad access to diverse data sources could enable Web3 AI agents to make more informed decisions and provide more comprehensive services.
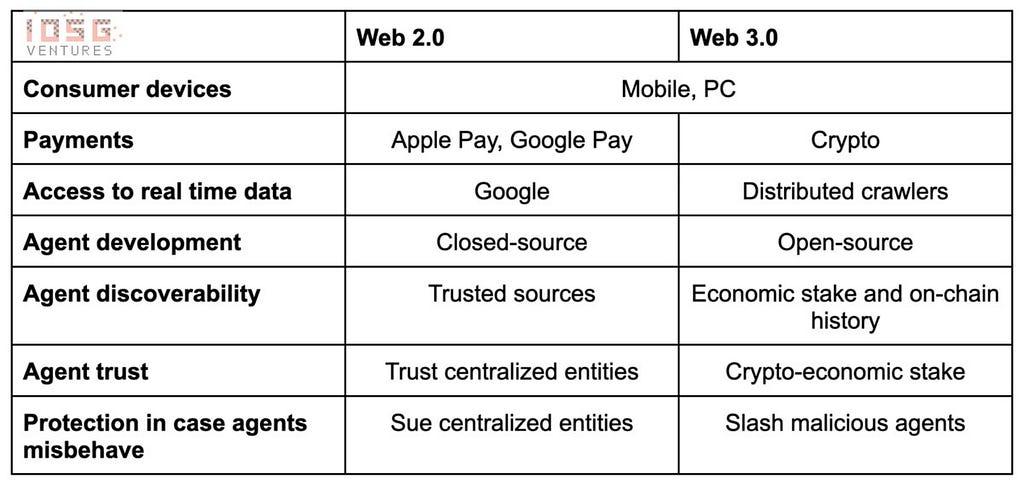
Limitations and Challenges for Web3 AI Agents
Limited Adoption of Crypto Payments
This post wouldn’t be complete if we don’t reflect on some of the adoption challenges Web 3.0 agents would face. The big elephant in the room is the fact that crypto still has limited adoption as a payment solution in the off-chain economy. Currently, there are few online platforms that accept crypto payments, which limits the practical use cases for crypto-based AI agents in the real economy. Without a deeper integration of crypto payment solutions with broader economy, the impact of Web 3.0 agents would remain constrained.
Transaction Scale
Another challenge is the scale of typical online consumer transactions. Many of these transactions involve relatively small amounts of money, which may not justify the need for trustless systems for most users. The average consumer might not see the value in using a decentralized AI agent for small, routine purchases if centralized alternatives exist.
Closing Thoughts
The reluctance of major tech companies to offer fully autonomous AI agents due to the unpredictable nature of non-deterministic models creates an opportunity for crypto startups. These startups can leverage open marketplaces and crypto-economic security to bridge the gap between the potential of AI agents and the practical concerns of implementation.
By utilizing blockchain technology and smart contracts, crypto AI agents could potentially offer a level of transparency and security that centralized systems would struggle to match. This could be particularly appealing for use cases that require high levels of trust or involve sensitive information.
In conclusion, while both Web2 and Web3 technologies offer pathways for AI agent development, each approach has its unique strengths and challenges. The future of AI agents may well depend on how effectively these technologies can be combined and refined to create reliable, trustworthy, and useful digital assistants. As the field evolves, we may see a convergence of Web2 and Web3 approaches, leveraging the strengths of each to create more powerful and versatile AI agents.

The Agent Wars: Silicon Valley Titans vs. Crypto Challengers was originally published in IOSG Ventures on Medium, where people are continuing the conversation by highlighting and responding to this story.